the Creative Commons Attribution 4.0 License.
the Creative Commons Attribution 4.0 License.
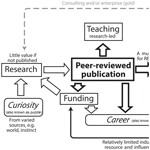
Demystifying academics to enhance university–business collaborations in environmental science
Geoffrey R. Saville
Mike J. Smith
Alister J. Scott
Emma K. Raven
Jonathon Gascoigne
Louise J. Slater
Nevil Quinn
Andreas Tsanakas
Claire Souch
Gregor C. Leckebusch
Neil Macdonald
Alice M. Milner
Jennifer Loxton
Rebecca Wilebore
Alexandra Collins
Colin MacKechnie
Jaqui Tweddle
Sarah Moller
MacKenzie Dove
Harry Langford
Jim Craig
In countries globally there is intense political interest in fostering effective university–business collaborations, but there has been scant attention devoted to exactly how an individual scientist's workload (i.e. specified tasks) and incentive structures (i.e. assessment criteria) may act as a key barrier to this. To investigate this an original, empirical dataset is derived from UK job specifications and promotion criteria, which distil universities' varied drivers into requirements upon academics. This work reveals the nature of the severe challenge posed by a heavily time-constrained culture; specifically, tension exists between opportunities presented by working with business and non-optional duties (e.g. administration and teaching). Thus, to justify the time to work with business, such work must inspire curiosity and facilitate future novel science in order to mitigate its conflict with the overriding imperative for academics to publish. It must also provide evidence of real-world changes (i.e. impact), and ideally other reportable outcomes (e.g. official status as a business' advisor), to feed back into the scientist's performance appraisals. Indicatively, amid 20–50 key duties, typical full-time scientists may be able to free up to 0.5 day per week for work with business. Thus specific, pragmatic actions, including short-term and time-efficient steps, are proposed in a “user guide” to help initiate and nurture a long-term collaboration between an early- to mid-career environmental scientist and a practitioner in the insurance sector. These actions are mapped back to a tailored typology of impact and a newly created representative set of appraisal criteria to explain how they may be effective, mutually beneficial and overcome barriers. Throughout, the focus is on environmental science, with illustrative detail provided through the example of natural hazard risk modelling in the insurance sector. However, a new conceptual model of academics' behaviour is developed, fusing perspectives from literature on academics' motivations and performance assessment, which we propose is internationally applicable and transferable between sectors. Sector-specific details (e.g. list of relevant impacts and user guide) may serve as templates for how people may act differently to work more effectively together.
- Article
(3386 KB) - Full-text XML
-
Supplement
(484 KB) - BibTeX
- EndNote
Political interest is increasing in converting research excellence into commercial success (e.g. Dowling, 2015; Evans, 2016; Mowrey and Nelson, 2004) and societal impact (e.g. Reed, 2018). Thus, the idea of the “entrepreneurial university” is gaining popularity (e.g. Etzkowitz, 2003; Slaughter and Leslie, 1997); it is argued both that universities might be fundamentally transforming into engines of economic growth (e.g. Feller, 1990; Florida and Cohen, 1999) or that there is a convergence to a hybrid where differences between scholarly and industrial activity become blurred (e.g. Owen-Smith, 2003). However, university–business collaborations could produce better outcomes through improved flow (also known as “diffusion”) of science innovation into policy and decision-making practices (e.g. Dowling, 2015; Rogers, 2003). This applies even in nations (e.g. UK, Australia) that rank relatively highly in the “Global Innovation Index” (Dowling, 2015; Dutta et al., 2017; Evans, 2016). So debate continues about how to incentivise, deliver, monitor and support such a change. This, and a political desire to see collaborations be more productive, is attested to by 14 reviews and studies in the UK on this topic in the last 12 years (see Dowling, 2015).
Effective university–business collaboration requires mutual understanding (e.g. Dowling, 2015), and developing this demands investment of time and effort. Scientists would benefit from a greater appreciation of business drivers, needs and constraints, and we propose that business (e.g. insurers) would be aided by understanding the answer to two questions. What motivates academics to do specific work? And, reciprocally, what might constrain them? By demystifying the motives of university scientists, this paper aims to make it easier to develop collaborations that are feasible and produce timely outputs, illustrated with a case study of the insurance sector.
In academic debate, models such as “diffusion” (e.g. Rogers, 2003; Scott et al., 2018; Ward et al., 2009) are used to understand how science may be better deployed in business and the cultural, institutional and individual barriers to this, but there is incomplete understanding of the exact nature of barriers facing academics, motivations to surmount them and coping strategies to do so.
D'Este and Perkmann (2011) review the recent literature on university–business interaction, including both informal (i.e. collaborative) modes (Grimple and Fier, 2010; Link et al., 2007) and more heavily studied routes (i.e. patenting, licensing and spin-off companies; e.g. Bercovitz and Feldman, 2006; Carayol, 2003; McMillan Group, 2016). Collaboration is the most frequent channel for interaction (D'Este and Patel, 2007; Perkmann and Walsh, 2007), including joint “pre-competitive” research that is often subsidised by public funding, heavily directed contract research of immediate business relevance and consulting. Regarding such collaboration, a small number of studies describe what academics' motivations are, compiling sizeable lists (e.g. Dowling, 2015). Studies delving deeper to understand these motivations are rare. For most UK academics, the driving incentive to interact with business is to further their research (D'Este and Perkmann, 2011). Drawing together three earlier concepts (e.g. Stephan and Levin, 1992), Lam (2011) divides motivations into “gold” (i.e. personal income), “puzzle” (i.e. knowledge and/or curiosity) and “ribbon” (i.e. funding and/or reputation), finding that a great majority of practicing university-based scientists are motivated by the latter two traditional rewards. The role of impact as a motivator has not been considered, and a good understanding has not been developed of why motivations may or may not be able to express themselves as actions.
As a mirror of motivations, it is possible to look at constraints upon academics. Like business, university science is a complex landscape, and the views, requirements and motivations of its actors (e.g. universities, individual academics and funding bodies) are not homogenous (e.g. Evans, 2016; Lam, 2011). Conventional wisdom suggests that intellectual property (IP) and cultural differences are key barriers to collaboration (Abreu et al., 2009; Lambert, 2003), and this is still borne out to some extent by studies such as Dowling (2015) that consulted a variety of stakeholders (e.g. universities, small and medium-sized enterprises, and trade associations) in which only 10 %–15 % of the input was from scientists themselves. Studies that consulted only university-based scientists as individuals (24 443 respondents in total), however, disagree strongly and rate these factors as relatively unimportant (Abreu et al., 2009; D'Este and Perkmann, 2011; Evans, 2016). These suggest limitations on time in a scientist's working day as an important (e.g. Evans, 2016; Lazarsfeld-Jensen and Morgan, 2009), and perhaps the overriding (Abreu et al., 2009), constraint on university–business collaboration. Speculatively this will dominate an academic's decision-making, framing actions whatever their motivations and desires may or may not be, but whilst this factor has been identified, it has not yet been explored in detail with respect to impact.
Part of generating viable university–business collaboration is that for work to actually proceed to the strategic and policy level, drivers must align with the incentive structures and constraints upon individual scientists and their motivations. These, sometimes conflicting, drivers are the main subject of this paper. The study's novelty is 3-fold. Firstly, direct and innovative data collection methods (Sect. 4) allow a broadly based (i.e. multi-university) and yet detailed view; consequently, a first synthesis in this context of academics' day-to-day duties and career-defining aspirational targets can be provided (Sect. 5.2, 5.3). Secondly, individual performance evaluation of academics has been only recently explicitly considered with respect to the research–teaching dipole (i.e. Cadez et al., 2017; Harland and Wald, 2018), and it fills a research gap by incorporating impact in this tensioned relationship. By investigating the day-to-day demands (i.e. micro-politics; e.g. McAreavey, 2006) upon an illustrative hypothetical scientist it gives insights into the pressures on their time (Sect. 6.1) and uses aspirational targets that govern their appraisal (Sect. 6.2) to add detailed and diagnostic understanding of why actions are prioritised. From this, a new conceptual model combining an academic's duties and motivations is developed (Fig. 4). Thirdly, pragmatic suggestions for specific actions to initiate and nurture a collaboration are proposed (Sect. 6.3). These are mapped back to appraisal criteria (Table 2) and impact typology (Sect. 6.2.2; Reed, 2018) to explain why actions may be effective, mutually beneficial and overcome the barriers that may be deterring scientists from working with business.
This work is differentiated by framing it for a business practitioner who engages with environmental science. Namely, the what and why that motivate university scientists are presented with the ultimate aim of conveying how a scientist might be pragmatically supported to effectively initiate and nurture collaborations with a business practitioner to the mutual benefit of both parties, in other words, to provide a user guide for practitioners. To this end, theory (e.g. Amsterdamska, 1990; Latour, 1987; Rogers, 2003) is kept brief, and specific examples are favoured over generalities where possible. One way it focuses is by only considering the varied (e.g. work–life balance, teaching and promotion) and multilevel (e.g. government and university) drivers as they affect the persona of a hypothetical illustrative environmental scientist with 10 years of faculty experience at a UK university (see Sect. 3). A second way is limiting illustrative, sector-specific detail to that for risk practitioners and (re)insurance.
Data and analysis are based within the environmental science discipline, but aspects of the analysis may be applicable more widely (e.g. social science and engineering) with caution, and the practical guide for risk practitioners in (re)insurance (i.e. Sect. 6.3) may serve as a template for how people may act differently to work more effectively together.
The insurance sector (see chap. 2.3 of Mitchell-Wallace et al., 2017) consists of entities that hold risk themselves (i.e. insurers, reinsurers and other financial institutions), companies who provide tools or advice to help them do so effectively (i.e. brokers, consultants and “vendor” model companies), and regulators. Natural hazards (e.g. flood, earthquake and tropical cyclone) present large risks; illustratively, USD 386 billion of damage accrued to insured assets alone in 2011 (Von Peter et al., 2012). Risk is commonly quantified using natural hazard risk assessment models called “catastrophe models” (see Mitchell-Wallace et al., 2017). Catastrophe models are vital in defining and implementing the financial mechanisms (e.g. reinsurance and catastrophe bonds) used to build resilience to the natural hazard risks, and there is a flow of peer-reviewed environmental science into them. However, inter-model differences exist (e.g. USD 13–72 billion for hurricane Maria in 1997; KCC, 2018). Thus, there is significant commercial interest in implementing the latest science to build the most realistic risk models. For example, the tendency of extra-tropical cyclones impacting Europe to cluster in time (i.e. occur in groups; e.g. Vitolo et al., 2009) has been included, and better understanding the tentative indicators that flood and wind damage tend to co-occur (e.g. Hillier et al., 2015; De Luca et al., 2017) is ranked as a current priority for this peril by insurers in a survey for the Lighthill Risk Network (Dixon et al., 2017).
With a few exceptions (e.g. Collette et al., 2010), much of the research and development to create risk models (e.g. RMS, AIR, SwissRe and JBA Risk Management) is undertaken in-house and typically applies selected knowledge from previously published peer-reviewed research, rather than generating new knowledge. This peer-reviewed environmental science is primarily generated in universities. However, quantifying the benefit of direct collaborations with university-based scientists (e.g. to make a business case) is non-trivial, especially as a company might have >50 % of technical staff qualified at the MSc or PhD level and commercial risk models are well established. More widely, in practice few (re)insurers feel that they have the ability to directly approach academia to question choices made about research applied in models or to keep abreast of the latest findings. Thus, a partial barrier to co-working and knowledge exchange (KE) directly between university scientists and practitioners (i.e. collaboration) exists, although its origins may be complex; further evidence for a partial barrier comes from the views of practitioners (Hillier et al., 2018). Challenges to collaboration vary by stakeholder (e.g. university, academic and business; e.g. Abreu et al., 2009; Dowling, 2015). For instance, business-relevant questions can be listed by insurers (Dixon et al., 2017; Lighthill Risk Network, 2016), but it can be hard to translate industrial needs into research questions that are precise enough for scientists to be able to answer and intriguing and novel enough for scientists to want to prioritise answering them.
The National Environmental Research Council (NERC), part of UK Research and Innovation (UKRI), sees environmental hazards as important, with an average investment of GBP 12.8 million per year in this area (Goff, 2015). Indeed, the insurance-sector and catastrophe modelling (i.e. of natural hazard risks) were highlighted in this 2015 report, with a number of recent initiatives directed at the insurance sector or accessible to it:
-
In 2017, NERC, DFID and ESRC combined to fund “Building resilience to natural disasters using financial instruments”, providing GBP 2 million.
-
In 2018 the Industrial Strategy Challenge Fund had a call entitled “Next Generation Services Research Programme” (Accountancy, Legal Services and Insurance), with a value of GBP 20 million.
-
Annually, NERC's “Innovation Placements” scheme provides ∼ GBP 1 million.
-
Over 5 years, the ongoing UKRI Future Leaders Fellowships are worth GBP 900 million.
Thus, both business and the university sector are seeking effective (i.e. mutually beneficial) pathways from the production of scientific knowledge in universities to pragmatic implementation, overcoming barriers. This being said, if insurance mirrors other UK business sectors, collaborations are “very patchy”, and, tentatively, there are some companies that are very active in building research collaborations with universities whilst a large number collaborate in a relatively restricted way (Dowling, 2015).
By better understanding what motivates academic researchers, it should be possible for business to forge synergies more readily with world-leading scientists. This will directly assist new and existing insurance-sector initiatives (e.g. the Willis Research Network and the AXA Research Fund) as they strive to access cutting-edge research to drive innovation most effectively, and it will also facilitate the building of interpersonal university–business relationships that are the key to effectively accessing government-funded initiatives (e.g. Dowling, 2015).
To focus this work and give specific insights, a model persona was created to represent an illustrative university-based environmental scientist. A typical university-based job including both research and teaching duties is assumed. Fellowships won in open competition (e.g. NERC and Royal Society) allow a scientist to focus on a stated work programme (e.g. in research or knowledge exchange), but they are prestigious, owing to their relative scarcity. Government-funded research centres exist (e.g. British Geological Survey in the UK), but staff are required to undertake applied and income-generating work alongside fundamental research. In some countries there are institutions intended purely for research (e.g. GFZ in Germany), but this is not so in the UK.
Our research scientist has 10 years of experience at the faculty level. This is regarded as early- to mid- career (e.g. https://www.egu.eu/ecs/, last access: 7 January 2019) and contains the transition from the lecturer (L) level to senior lecturer (SL) level in the UK system; permanent positions (i.e. not fixed-term contracts) typically start at L or “assistant professor” then progress through SL or “associate professor” and “reader” or “associate professor” to “professor” (e.g. Broch et al., 2017). This stage is ideal for having established a research track record yet still being flexible and actively seeking to initiate new long-term relationships. Thus, it is a type of person who, whilst not yet deeply engaged in KE, a risk practitioner is perhaps most likely to encounter and want to initiate and nurture a long-term relationship with.
Critically, this hypothetical individual's core research and scientific identity involves improving understanding of physical processes (e.g. physics, atmospheric science, geology and hydrology). As such, they can likely make a genuine contribution to risk assessment models for natural hazards. Also importantly, this scientist is assumed to have a genuine and significant interest in impact (i.e. real-world change; see also Sect. 6.2.2), working directly with business, and has at least some work that is of interest to insurance or reinsurance in natural hazards. Their level of experience in KE could vary, depending on background (e.g. KE Fellowship, grants or worked in business), and they may or may not have done reading (e.g. Reed, 2018) or training in impact. Thus, whilst they are primarily judged on scientific research, and KE cannot be their core business, it is assumed that any barrier here is in factors (e.g. KE skills or time) other than willingness to try.
Our research scientist is assumed to be effective, efficient and hardworking, and to have a desire for both a successful career continuing with their university and work–life balance. At work, in line with the great majority of academics, this person is motivated by career (i.e. funding and/or reputation) but ultimately by the puzzle (i.e. knowledge and/or curiosity; Lam, 2011; Stephan and Levin, 1992; see Sect. 1). If they were more interested in the gold, they may start a spin-off company rather than engaging in the type of joint collaboration considered here, for example.
Finally, it is assumed that this person is in reasonably good mental health, although mental health is a serious issue in the sector with a notable self-reported negative impact of work for over half of UK academics (Bothwell, 2018), and is assumed to be a “rational actor” that in the main responds logically to their internal and external motivations and drivers (e.g. performance appraisals; Cadez et al., 2017; Grendon, 2008; Harland and Wald, 2018; Moya et al., 2015).
4.1 Methods
The persona of a typical, impact-inclined, early- to mid-career UK academic (see Sect. 3) was used to focus and constrain the scope of the work. This approach is innovative and is an effective mechanism for achieving the study's aims, in particular ultimately deriving specific guidance for practitioners. The overall approach draws upon ideas of reflexivity (e.g. Bostrom et al., 2017) and action research (e.g. Denscombe, 2010; Kemmis et al., 2013), i.e. academics and practitioners considering their work environments and participating together to solve a problem to produce guidelines for effective practice. Within this, a mixed-method approach was used to meld objective data with the knowledge and experience of two cohorts of university-based environmental scientists, effectively assimilating a diversity of views of, and external drivers for, teaching, research and engagement with business. Three sources were used to create the evidential base for this work:
-
Freely available textual data (i.e. job specifications and promotion criteria). These present a university's preconsidered distillation of requirements and aspirations, from multilevel and varied internal and external drivers (e.g. student expectations or national government policy) against which a UK academic will typically be assessed; these may deviate from actual practice. This is a new approach in this area of research.
-
The first-hand experience of a cohort of 17 academics (environmental scientists) and five business-based co-authors. This is a direct bridge to actual practice and is therefore complementary and unmediated (i.e. no interposed social scientist). These data provide a view of tasks and criteria filtered through the perception of university-based environmental scientists and are thus biased. However, the bias is appropriate for this study; perceptions of tasks and criteria are illuminating when forming an understanding of the motives of those doing the perceiving. Whilst this is an innovative form of data collection, working as co-authors is valid and appropriate in this particular instance, as this is a familiar, natural and pragmatic mode of engagement for these contributors. Unlike participants in most studies, a pre-existing document is no barrier to offering criticisms and suggesting changes – indeed, quite the opposite is typical. Where doubt existed with respect to comments, three semi-structured interviews were used to clarify meanings. Co-authors were selected by two means: (i) on the basis of likely interest in the research from Hillier's network and (ii) by volunteers from a list of attendees distributed well in advance of the workshop (see below).
-
A workshop at NERC's Knowledge Exchange Network (KEN) meeting, 26 June 2018 in Glasgow. The session analysed the textual data. Six participants were from business. The 21 university-based environmental scientists comprised seven faculty (i.e. permanent contracts), 11 post-doctoral researchers and one PhD student, with varying levels of industrial experience. Eleven participants are also co-authors.
In total, even while only taking participants and co-authors' current institutions into account, data pertaining to 36 of the UK's 164 universities were collected. Details of the methodology are given below with respect to the two questions central to this investigation. What motivates academics to do specific work? And, reciprocally, what might constrain them?
Table 1Illustrative expectations (i.e. the duties) of a typical early- to mid-career UK academic, based on a thematic analysis. Numbers in brackets (e.g. [7]) indicate the number of occurrences within 10 job specifications. Square brackets [] are for analysis by the lead author, and curved brackets () are from the workshop, which agree well (i.e. R2=0.77). Italic text distinguishes items not in the majority of job specifications. Underlining denotes the subset of three research tasks related to a scientist's own hands-on work (see Fig. 3).
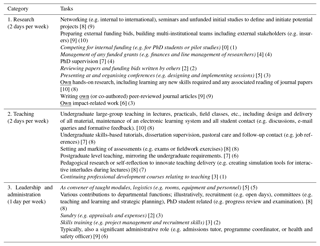
4.1.1 Investigating time as a primary constraint
A primary constraint upon collaborations is self-reportedly the time available in an academic's working week (e.g. Abreu et al., 2009; see above). Thus, a pertinent question is the following: what do research scientists in universities do? More specifically, what are the duties and responsibilities of a scientist? What are they required to do? Data to answer these provide necessary context for understanding competing pressures placed upon them, as these day-to-day tasks frame what a scientist can do, whatever their underlying desires and motives may or may not be.
Thematic analysis (e.g. Dowling, 2015) was used to build a list of representative, illustrative expectations from the detailed specifications in 10 job advertisements (Table 1). Initial review was by Hillier, with Table 1 updated and adapted in light of two rounds of comments from the 17 academic co-authors before the workshop; experience-based context and caveats surrounding Table 1 in Sect. 5.2 are a synthesis of these comments. Finally, at the workshop, participants rated the statement “Table 1 is, on balance a fair representation of demands on a UK academic” using a five-point Likert scale. Thus, duties in Table 1 are derived with the intention of providing a fair level of comparison with expectations within specifications in their number and scope, although some are amalgamated or split here when compared to individual job profiles.
As a cross-check, participants at the workshop also replicated Hillier's assessment of how often each point in Table 1 was explicitly present in each job specification. The 10 groups of two to three participants had one specification and were instructed to interpret “explicitly” as they wished.
Arguably, whilst giving a university's considered view on requirements, the tasks in job specifications may deviate from actual practice. Both the workshop and use of 17 co-authors mitigate this limitation and allow a legitimate view on it to be given.
To obtain 10 job advertisements, a non-exhaustive search protocol was used, but it was one that effectively offers random and objective selection with respect to the information sought; specifically, the advertisements used are the first 10 hits from a device located in the UK for the search “job description university lecturer” on the Google search engine on 16 May 2018. Only job specifications for advertised posts taken directly from universities' sites were used (i.e. not agencies or career advice sites). Taking the search results in descending order, 18 were required to find 10 such results. Ten is a relatively small sample of 164 UK universities, but it is sufficient for key themes to robustly emerge (see Table 1).
Table 2Indicative set of appraisal criteria for an early- to mid-career research scientist based in a UK university, as distilled from promotion criteria to senior lecturer in the context of co-authors and workshop participants' experience. Percentages indicate the relative occurrence of the categories as main headings within the publicly available promotion criteria.
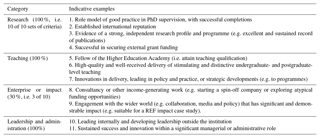
4.1.2 Investigating performance appraisal as a substantive motivator
A second area of interest is how research scientists in universities are motivated and, in particular, how this might be influenced by how they are assessed in their employment. Appraisals (e.g. annual performance review) are now ubiquitous in universities (e.g. Costa and Olivera, 2012; Su and Baird, 2017). Appraisal criteria pertain to strategic aims and aspirations of each university distilled into a form applicable to individuals, and they are typically designed to motivate an academic to develop in their job role (i.e. towards promotion), although an element of judgement is common (e.g. a classified outcome such as “very good” might be given). As such, the co-authors' experience is that appraisal criteria are strongly aligned with promotion criteria, at least sufficiently in that, with an appropriate process, an indicative set of appraisal indicators may be derived from promotion ones. These indicative appraisal criteria then provide a useful semi-objective basis (i.e. Table 2) for understanding academic motivations. The last step in the analysis is necessary, as indicative appraisal criteria are not typically public domain, whilst promotion criteria commonly are in the UK. These criteria that reflect a longer timescale and differ distinctly from tasks at the day-to-day (i.e. operational) level are noted in Table 1.
For Sect. 5.3 thematic analysis was used to build a list of representative and illustrative appraisal criteria from currently applied, freely available guidance on promotion to senior lecturer (see Sect. 3) from 10 UK institutions (Table 2). Initial analysis was by Hillier, with Table 2 reviewed and adapted in light of two rounds of comments from the 17 academic co-authors before the workshop; experience-based context and caveats surrounding Table 2 are a synthesis of these comments.
Word clouds were generated to assist understanding; Fig. 1 contains all relevant texts from the specifications, whilst Fig. 2 contains words perceived as significant by the workshop participants. Participants considering a set of criteria highlighted one to five snippets of less than five words in each of the four main areas (i.e. research, teaching, enterprise or impact, and leadership and/or administration). Whenever categorisation in the documentation was different from the main area in Table 2 (e.g. “managing people” or “pastoral care”), participants judged which of the four areas to identify the contents with.
UK promotion criteria were obtained from all relevant hits of the 190 returned for a search of “university academic appraisal criteria” on the Google search engine on 1 May 2018 using a device located in the UK.
4.1.3 Pragmatic suggestions for collaboration
The ultimate aim of this work is to suggest how an environmental scientist might be pragmatically supported to collaborate effectively with business practitioners. Views here are based on the personal experience of all 22 co-authors.
4.2 Limitations and biases
In addition to the limitations and biases discussed above, two others exist but do not invalidate the work.
-
Bias to the subset of environmental scientists participating in this research, i.e. participants and co-authors, are inclined towards knowledge exchange (KE). This limitation is accepted, but this is the scope of the study (i.e. experience broadly aligns with the persona used.)
-
Focus on an illustrative academic persona (Sect. 3). This precludes considering all variants, but sets a basis for future studies.
Many potential avenues are not explored, e.g. KE for social scientists, where understanding the processes of relationship building and better collaboration can be “core business”. Equally, a full-scale guide for academics to their business-sector partner is out of scope.
4.3 Ethics
Data collected at the workshop were undertaken in accordance with good practice, and clearance was given by Loughborough University's departmental ethics coordinator. Contributors to the paper were under no obligation to become co-authors.
5.1 Hours worked
Faculty level participants at the workshop self-reportedly work a mean of 47.9 h per week, ranging from 38–70 h, normalised to one full-time equivalent (FTE) where they work part time. They worked during 18.3 weekends per year on average, with a range from 3–40 weekends. These data for UK university-based environmental scientists with an inclination for KE are broadly consistent with the experience and practice of the 17 academic co-authors.
The result gives a view on the spare capacity within an academic's typical week, so it is pertinent when considering time pressure as a constraint on collaboration (i.e. in Sect. 6.1.1).
5.2 Duties of research scientists in universities
Table 1 illustrates the main duties expected of a typical UK university-based early- to mid-career environmental scientist, namely with 10 years of faculty experience. There are 22 tasks based on thematic analysis, roughly commensurate with the median of 28 “key” or “main” duties and responsibilities in the job specifications analysed; the range is 15–52 tasks. The consensus of the 17 academic co-authors is that Table 1, including the time allocation, broadly reflects our experience in UK universities. Similarly, in the workshop, more than twice as many participants agreed as disagreed (12 vs. 5) with the statement that “Table 1 is, on balance a fair representation of demands on a UK academic”. Notable details from the table and experience-based caveats of the academic co-authors to it are reported below, including extra detail from the original job specifications where it is useful.
Teaching will readily expand beyond 2 days per week on average if permitted to by the researcher, as will administrative duties, and this load is spread unevenly throughout the year; it is common for little research (including impact) to be possible in term times, with a real chance that none is possible for 1–2 months during a busy term (i.e. if an imbalance in teaching load between terms exists). This effect becomes severe if programmes or modules need to be rewritten or restructured, which can take hundreds of hours whilst other demands do not lessen.
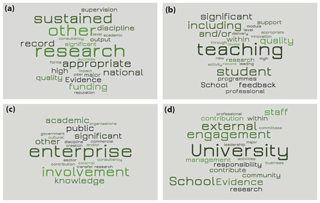
Figure 1Word clouds summarising promotion criteria for 10 UK universities at senior-lecturer level in the four main assessment headings: (a) research, (b) teaching, (c) enterprise or impact, and (d) leadership and administration. Minimum frequencies vary from two to five to give 20–30 words displayed. Sizes according to rank.
Duties occurring in most job specifications (normal typeface in Table 1) are all time-consuming requirements. However, in the experience of the academic co-authors, those with low numbers of occurrences are also ubiquitous (e.g. reviewing funding bids and papers written by others) and illustrate the numerous other activities a researcher is simply expected to find time for (e.g. Lazarsfeld-Jensen and Morgan, 2009). Several activities to show leadership outside the university are also usually required. Examples of such roles include journal editing, sitting on panels assessing funding bids, treasurer for learned societies (e.g. British Society for Geomorphology), external examiner at other universities, sitting on government committees, working with funding bodies to define future research directions and outreach (e.g. Pint of Science https://pintofscience.co.uk/, last access: 7 January 2019). Note that a scientist's own, hands-on research activity (i.e. doing it rather than managing it) forms a relatively small part of the 2 day per week allocated to research, and impact (also known as innovation, consultancy or KE) is only a part of this.
Professors and readers are also usually expected to undertake more substantive management roles (e.g. head of department, lead of a doctoral training centre, admissions tutor or programme director). Other requirements are sometimes reduced to account for such time commitments, and the workload models used to measure and allocate such activities are often controversial (e.g. Lazarsfeld-Jensen and Morgan, 2009).
These day-to-day tasks show that scientists have an array of competing demands for their time, so they frame what a typical university-based scientist can do, whatever their underlying desires and motives may or may not be. In summary, a highly time-limited environment is indicated, as discussed in Sect. 6.1.1. Thus, these results provide the necessary context to understand what specific work our illustrative scientist will or will not be able to do and why (see Sect. 6).
5.3 Criteria used to assess research scientists in universities
Table 2 is an indicative set of appraisal criteria for an early- to mid-career UK academic, derived from thematic analysis of promotion criteria to senior lecturer. On balance, and taken as an illustrative realisation of a more complex totality, these are a fair representation of criteria used to frame yearly appraisals in the experience of the 17 academic co-authors.
Of the four main areas (i.e. research – R, teaching – T, enterprise or impact – E/I, and leadership and administration – L/A), all but E/I are always present as a main heading within the criteria (Table 2). E/I is a main heading in only three of the 10 institutions (30 %), although detailed examination of the documents reveals that criteria relating to E/I are pervasively present in all UK institutions. This is consistent with the knowledge and experience of the 17 academic co-authors.
To be viewed as acceptably meeting expectations, good performance in at least two of the three traditional categories (i.e. R, T and L/A) is typically required in the UK; this is based on co-authors' experience and examination of the criteria documents. Word clouds below directly display an impression of key aims from the underlying text (Fig. 1) and how the aims were perceived by academics at the workshop (Fig. 2). Notable elements of the word clouds are summarised in the sections below (Sect. 5.3.1–5.3.4), accompanied by explanation based on the co-authors' experience and reading of the underlying texts; a summary of the aspirational criteria used to assess UK academics precedes this.
In short, publishing novel science in peer-reviewed journals is the overriding imperative, followed by winning funding to facilitate publications (i.e. by funding a post-doctoral researcher). Teaching and leadership and administration are obligatory. Pervasive pressure (i.e. criteria) exists to undertake enterprise or impact work, in whichever diverse form, but in practice it remains lower in priority, is not usually obligatory and is best engaged in if reportable outcomes are also aligned with other drivers.
Thus, these results give an indicator of how our illustrative scientist may respond (e.g. in terms of prioritisation) to time pressure within the work context in the presence of impact requirements, discussed in Sect. 6.1.1, and direction from funding bodies, discussed in Sect. 6.1.2.
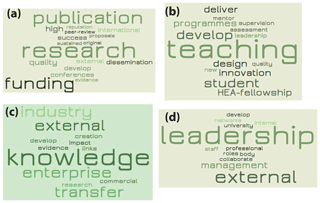
Figure 2Word clouds summarising the workshop participants' perceptions of promotion criteria for 10 UK universities at senior lecturer level in the four main assessment headings: (a) research, (b) teaching, (c) enterprise or impact, and (d) leadership and administration. Minimum frequencies of two. Sizes according to frequency.
5.3.1 Research
Key words for promotion to senior lecturer show the need for a sustained high-quality research records (i.e. publications) and funding (Fig. 1a). Academics' perceptions focus on these even more dramatically (Fig. 2a). These are again repeated in our illustrative, representative profile (Table 2), but this also includes an emphasis on PhD supervision and reputation. These may appear disparate, but in the experience of the academic co-authors are strongly bound together.
A university scientist's international reputation is built almost entirely on novel, high-quality, well-cited peer-reviewed publications (i.e. journal papers); these evidence a research profile, and incomplete (i.e. unpublished) work is of little value. Funding bids must be underpinned by related publications, with some flexibility to take moderate steps in new directions driven by curiosity, and it provides the resources (e.g. post-doctoral researchers) to create excellent publications. Whilst PhD students' development and needs are paramount in their supervision, a well-supported student can often (i.e. is typically well advised to) co-publish with their supervisor, which is also to that academic's benefit in a university environment where time to conduct their own hands-on research is limited.
5.3.2 Teaching
Key words for promotion to senior lecturer show the need for significant student-focussed teaching of quality (Fig. 1b). Academics' perceptions also include an emphasis on development, design or innovation, and a Higher Education Academy (HEA) Fellowship (HEA-Fellowship) is present (Fig. 2b). The illustrative, representative profile (Table 2) adds context, such as for the HEA-Fellowship; in the UK a professional qualification with a body such as the HEA is required to evidence attainment in teaching. Also, Table 2 explains “develop”; this could be of new module (e.g. a set of 10 lectures and practical sessions) or programmes (e.g. a new “Global Environmental Risk” BSc), although there is an expectation of innovative and stimulating modes of delivery (e.g. experiential, problem-based learning and integrating tablets). In the experience of the academic co-authors, student satisfaction is important in practice, as measured internally by module or programme feedback and externally by the National Student Survey (NSS, https://www.thestudentsurvey.com/, last access: 7 January 2019).
“Research-led” teaching based upon a scientist's core research is required, but the teaching does not feed back into the scientific research. However, teaching is typically the main source of university funding (Universities UK, 2016) and is monitored in the national Teaching Excellence Framework (TEF) assessment (https://www.officeforstudents.org.uk/advice-and-guidance/teaching/what-is-the-tef/, last access: 7 January 2019), so this is not typically optional (see Sect. 5.2). The expectation is to design, maintain and deliver customised material that exceeds that found in textbooks, increasingly based on the academic's own published interests as a degree proceeds.
5.3.3 Enterprise or impact
Enterprise occurs frequently as a key word within promotion criteria for senior lecturer (Fig. 1c), but neither academics' perceptions from the workshop (Fig. 2c) nor our illustrative, representative profile (Table 2) are able to focus on specifics. Examination of the underlying criteria and words used to generate the clouds show that this is due to the range of possible activities here.
The academic co-authors' experience indicates that, whilst of increasing importance, enterprise or impact activity is only considered of value if it generates income to fund future research or is suitable for a Research Excellence Framework (i.e. REF, https://re.ukri.org/research/research-excellence-framework-ref/, last access: 7 January 2019) impact case study and ideally facilitates or inspires better curiosity-led research. This being said, pressure to engage in impact-related work is pervasive in the UK from institutions and funders.
5.3.4 Leadership and administration
Key words for promotion to senior lecturer show the need for evidence of contributions to the department or school and university (Fig. 1d), whilst academics' perceptions highlight that this includes leadership and leading externally (e.g. driving national and international initiatives or promoting a university's brand; Fig. 2d). The descriptors in our illustrative, representative profile (Table 2) are more explicit, stressing leadership, success and innovation. Outreach (e.g. public talks and sixth-form summer research experience) is encouraged, but is essentially optional, and delivery of all standard administrative tasks (e.g. research team management and undergraduate module leadership) is taken as read. L/A will not get an academic shortlisted for a job or promotion, but evidence of competence in this is required for them to actually get it.
Successful university–business collaboration requires mutual understanding, built upon shared vision and long-term trust-based personal relationships (e.g. Dowling, 2015). In building collaborations, business practitioners will be assisted by understanding the answer to two questions. What motivates academics to do specific work? And, reciprocally, what might constrain them? Specifically, this work adds insight into why motivations arise and how exactly time constraints manifest themselves in behaviours in the presence of impact requirements. This discussion provides a window into the motives of university-based research scientists that, in addition to practitioners, will be highly relevant to a number of academic colleagues, university administrators and policymakers.
Constraints on collaborations are considered first, in Sect. 6.1. Then, environmental scientists' motivations are discussed in Sect. 6.2, culminating in an improved conceptual model of academics' motivations (Fig. 4). Section 6.3 considers the practical aspects of building a university–business collaboration based on a 1-to-1 relationship, with a focus on environmental science and the insurance sector; an illustrative, non-exhaustive list is proposed of pragmatic suggestions for short-term and time-efficient activities that have reportable and mutually beneficial outputs for both an academic and a risk practitioner in order to build the long-term trusting relationship needed for collaboration.
6.1 What constrains scientists when working with business?
Two-thirds or more of university scientists have a prime interest in research rather than teaching (Abreu et al., 2009; Cavalli and Moscati, 2010). However, teaching-related work is not optional (Sect. 5.3). This leads to a conflict for limited time between teaching and research (Sect. 5.3), which is widely reported (Arnold, 2008; Gendron, 2008; Harland and Wald, 2018; Moya et al., 2015), even if the consequences of this continue to be debated (see the summary in Cadez et al., 2017). For an environmental scientist, work with business is based upon their science, and this study shows that for workload purposes, this typically falls within time allocated to research (Table 1). This section expands the debate by incorporating impact into this tensioned research–teaching relationship. Specifically, it considers the influence of time pressure due to workload factors (Sect. 6.1.1), the role of funders (Sect. 6.1.2), intellectual property (Sect. 6.1.3) and academics' need for a coherent track record (Sect. 6.1.4) as potential constraints upon university–business collaboration.
6.1.1 Time pressure
A self-reported mean of 47.9 h per week is worked by the sample of UK-based environmental scientists involved in knowledge exchange (Sect. 5.1), which is consistent with larger studies. The Changing Academic Profession (CAP) survey of 100 academics (2004 to 2012; Teichler et al., 2013) describes a self-reported mean workload of ∼48 h per week, with 45–50 h per week in the UK, and is supported by recent data from 2000 UK academics (Bothwell, 2018). This time at work sets the boundary conditions for accomplishing the 15 to 52 distinct key or main tasks required of a university-based scientist, in addition to which there is an expectation to do numerous other tasks to support their academic reputation, internal visibility and external profile (Sect. 5.2). It is also the case that UK academics often work at weekends yet feel under pressure to do more (Sect. 5.1 and 5.2; Bothwell, 2018). Thus, even working ∼50 h per week, it is evident that there is time pressure for a typical university-based scientist in the UK; i.e. there is no spare (i.e. previously unallocated) time. With the presence of time-pressure established, it is now useful to proceed on the temporary assumption that any impact-related work will be substituted into an academic's typical hours and consider what fraction of time might be made available for collaborations with business.
Table 1 reports a ratio of approximately for in the UK, with the nature of the need to juggle these demands described in Sect. 5.2. These ratios are entirely consistent with the CAP survey (Teichler et al., 2013), making Table 1 widely relevant. The ratios also align with the co-authors' view of the UK system, giving ∼2 days per week for all research-related work although, critically, in addition, the introduction of enterprise or impact (Tables 1 and 2) and pervasive pressure to act on this (Sect. 5.3) has increased the difficulty of the time-management challenge amid competing demands.
Table 1 adds clarity to what is covered by research within the categorisation used here. Specifically, it includes impact-related work as well as numerous other tasks (e.g. PhD supervision, preparing funding bids and grant-related administration) that might not immediately be thought of by business practitioners when considering an academic doing research. Each of these 10 illustrative main duties is multifaceted. For instance, their own hands-on research conducted by the academic includes elements such as reading articles, modelling, programming, learning any new skills required and writing journal articles. We do not argue that this categorisation is the only one possible or that the tasks itemised are prescriptive. Indeed, a number of alternative tasks that could be prioritised and substituted in for any individual academic are reported in Sect. 5.2. However, the view of the 17 academic co-authors and workshop participants is that Table 1, distilled from job specifications, is on balance a fair representation of the demands on a UK academic. To wit, key elements are present, and the number and magnitude of tasks form a suitable basis for an evidence-based, indicative view of time that might be available for impact-based work.
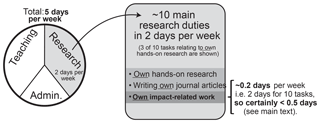
Figure 3Potential time availability for collaboration with business, in the context of other duties, of a typical early- to mid-career UK academic. Time available is divided between teaching, research and administration (; see main text), and then an academic's own hands-on research, writing and impact-related work are only three of 10 tasks within research, giving 0.2 days per week for each task, assuming an equal distribution.
Figure 3 summarises the logic behind an estimate of up to 0.5 days per week. A ratio of for leads to 2 days per week for research. Then, an equal distribution of time between the 10 tasks within this category implies ∼0.2 days per week for impact-based work. This is moderated by the knowledge and experience of the 17 academic co-authors, assimilating the relative priority that must be given to the tasks in light of assessment criteria (Sect. 5.3). Without some special circumstances to buy out an academic's time (e.g. KE Fellowship), for some co-authors 1 h was a limit, and not even that in term time. The experience of others is that, with determination, it is possible to preserve 1 day per week for the totality of the three tasks related to hands-on research (see Fig. 3). Ultimately, the co-authors' consensus is that, if strongly prioritised (i.e. intermediate term benefits clearly identified), 0.5 days per week was a ceiling to what might be possible. This being said, readers can review the evidence (e.g. Table 1, Fig. 3) and form their own view.
Recent data (2016–2017) show that 33.1 % of UK academic staff are part time (HESA, 2018). Consideration of additional issues surrounding part-time contracts is beyond the scope of this work, but it is expected that responsibilities will reduce commensurately with reduced hours. If the range of responsibilities is reduced, and the challenge of non-scalable tasks (e.g. yearly appraisal) is tackled, time to collaborate with business may be similar to full-time staff. However, if time is simply spread more thinly, the potential time to collaborate will be reduced accordingly.
In short, the critical new observation is an estimate that an efficient and effective full-time academic might retain about 1 day per week in which to do their own hands-on research; of this up to 0.5 days could be committed to business-focussed (e.g. insurance sector) implementation (i.e. impact) without case-specific negotiation (e.g. for a KE Fellowship). Prioritisation is key, and without expanding working hours, any choice to do something is inevitably a choice to discard an opportunity of less potential value; often, an impact task will be competing directly against the little curiosity-driven research that is possible. A convincing (self-)justification is therefore likely needed well before any official appraisal. With any expansion of hours, impact-related work could readily be competing against time with family, children or weekend recreational activities (e.g. Bothwell, 2018).
As Teichler et al. (2013:99) note, the presence of stringent time constraints is certainly not unique and applies equally to other high-skilled jobs (e.g. in the insurance sector). So, taking a positive view, mutual understanding of the nature of each other's time-pressured work environments might be a point of commonality and help in building trust-based personal relationships between academics and practitioners within business.
6.1.2 Direction from funding bodies
Ultimately, the topics, scope and even existence of environmental science research are set by funding bodies. Funders may be government (i.e. UKRI and NERC) or business (e.g. the Willis Research Network and AXA Research Fund). Government funding may be either specific to particular projects through grant bids or to particular funding “calls”, with eligibility potentially restricted to certain topic areas, geographic locations or the status of the applicant. Conversely, if a business is prepared to fund a particular topic, then there is a good chance it will get done. It is also notable that UKRI funding now typically incentivises collaboration with business, discussed below (Sect. 6.2.2).
6.1.3 Intellectual property
Dowling (2015, p. 27) review the barriers to university–business collaboration (e.g. identifying partners inside insurers; Abreu et al., 2009). One notable barrier comes from any rigidity in expectations about knowledge ownership and exclusive use (e.g. Dowling, 2015). If expectations are too strict, this presents a fundamental mismatch between creating benefit for society directly by publishing (i.e. not supporting the profits of one company) and supporting the public only indirectly via tax of increased revenue or better (re)insurance products. Ultimately, university-based environmental scientists cannot compromise on publication. Illustratively, a compromise is to use post-project embargo periods for publications or data (e.g. 6–12 months; e.g. Drexl, 2016; Morris et al., 2011; Moulin, 2018; UKRI, 2018b), perhaps explaining relatively low levels of concern amongst individual academics about this issue (i.e. Abreu et al., 2009). The insurance sector is a multinational illustration that these considerations are similar across the globe but also that solutions exist (see below).
6.1.4 Track record
Globally, international reputation is important both directly in appraisals (e.g. Table 2) and obtaining funding and requires a track record in specific activities (Sect. 5.3.1). Thus, there is some need for continuity in research themes, which may be perceived as a pursuit of “pet projects” by business; this term voices the frustration felt in business when an academic appears to use a collaboration as a vehicle for pursuing an existing blue-skies project without deviation to accommodate business needs.
6.2 What motivates research scientists to do specific work?
The majority of new knowledge that could be used by business (e.g. insurance sector – see Sect. 2) is published by university-based scientists in journals. How can practitioners best access and harness this existing knowledge and work with these researchers to answer new questions as they arise? With basic needs met (see Olsen, 2004), additional personal financial reward (i.e. gold) is of low importance to the great majority of researchers (e.g. Abreu et al., 2009; D'Este and Perkmann, 2011; Evans, 2016; Lam, 2011), who do little or no consultancy work; so, for a risk practitioner, it does not matter how much you might be able to pay them to work with you. Persuading the world's best researchers to work with you requires a deeper understanding of what motivates most academic researchers, so this section considers why academics' motivations arise and what governs their relative dominance.
The findings of this study (Sect. 5), and the experience of the co-authors, leads us to propose impact as a notable addition to prior models (e.g. Lam, 2011; see Sect. 1). Broadly speaking, therefore, after gold, three types of interlinked motivations remain (i.e. Fig. 4) that influence our illustrative research scientist. Each of these presents an opportunity for a risk practitioner.
-
Curiosity and creativity (also known as puzzle; Lam, 2011). By temperament, given unlimited time and funding, academics would simply study whatever interests them most for the satisfaction of a puzzle solved in an innovative way. How can you frame your needs in a way that will pique the curiosity of researchers, challenge them and give them opportunities to conduct creative, original and publishable work?
-
Impact. Some academic researchers want to make a positive impact upon society (Reed, 2018; i.e. “altruism”), whilst others are intrinsically motivated by the act of working with business itself (i.e. “utility”). How will working with your company give these researchers a unique opportunity to make a difference that is significant, meaningful and at a scale not otherwise possible?
-
Career (also known as ribbon; Lam, 2011). Increasingly, generating such benefit in the real world is now rewarded, with some contribution to winning research funding and promotion (see Sect. 5.3). How can you provide evidence of impact from research that can be used by researchers in evaluation exercises?
These drivers are considered below.
6.2.1 Curiosity and creativity
Curiosity is a major driver for most researchers (e.g. Lam, 2011), who want to be at the cutting edge of their discipline. The excitement of discovering something new can be addictive, even when the breakthrough seems elusive, and many researchers are motivated by the intellectual endeavour required to overcome the challenges that stand in their way. Sometimes the journey is as rewarding as the destination, as researchers are forced to engage with new disciplines and ways of thinking in their pursuit of creative solutions. Also, the challenge of coming up with new solutions to old problems should not be forgotten.
The findings of this study (Sect. 5) in no way contradict existing views of creativity and curiosity. Fundamentally, curiosity is the seed from which all academic publications grow, and publications remain central to international academic reputation and appraisal (see Sect. 6.2.3). However, the results reveal the bounds (e.g. time around other duties) in which curiosity must operate. This effectively limits the utility of vague, unconstrained or highly speculative curiosity; such tasks are unlikely to rise to the top of a list of pending actions. It is therefore important to focus and formulate questions that are precise enough for the scientist to be able to answer, and intriguing and novel enough for scientists to want to prioritise answering them.
So, how can a practitioner (e.g. in insurance sector) tap into this set of motives?
-
Embrace complexity. Rather than simplifying the nature of the challenges you need to address, can you explore the complexity of the challenge and ask why questions that cultivate your own sense of curiosity in the challenge as something to be understood, not just solved.
-
Articulate what is not known. Before engaging with academic researchers, have you checked that there is not already an answer to your question in the research literature? Google Scholar has made it easier than ever before to access published research. Use what you learn from your reading to put your question into the context of what is already known and explicitly articulate what is not yet known; this is an ideal way of both identifying an academic researcher and framing your approach to them. Alternatively, if time or access to publication is a barrier, you might engage with an academic so as to use such a scoping exercise as a mechanism for collaboration building; detail on useful approaches to this is in Sect. 6.3.
-
Consider opportunities. Consider what unique opportunities you can give to a researcher who loves the creativity of what they do. Can you expose them to new ways of working or thinking, introduce them to colleagues who ask challenging questions, or expose them to methods used in the business world to drive original thinking and innovation?
-
Facilitate collaboration between research fields. Actively promote (e.g. host events or provide needs-based rationale to pursue) multi-sector collaboration, which opens new avenues for innovative research (e.g. across traditional subject boundaries).
6.2.2 Impact
Impact is a term used to describe the influence that underlying research has outside academia (Reed, 2018). In the UK government bodies, i.e. NERC and HEFCE (HEFCE, 2015), which have now merged into UKRI (https://www.ukri.org/, last access: 7 January 2019), define impact broadly as “an effect on, change or benefit to the economy, society, culture public policy or services, health, the environment or quality of life”, although definitions vary in detail (e.g. https://www.ukri.org/innovation/excellence-with-impact/, last access: 7 January 2019)
Some academic researchers, especially in applied disciplines such as environmental science, have trained because they intrinsically want to make a positive impact upon society (Reed, 2018), i.e. altruism. Alternatively, we assert that others, including a group of the co-authors, are motivated by the act of working with business itself, assisting pragmatic implementation and being useful in that way; illustratively hearing “we can use this” or “that would be really valuable” energises these academics. This may be dubbed utility. Whatever a scientist's exact internal motivations, however, the findings of this study (Sect. 5) highlight that impact work must align with other demands of time, such as research and teaching, that are currently considered more important for the role of an academic and for promotion; this is despite the recognition of impact in job descriptions and promotion criteria.
This research also demonstrates that for a research scientist's job, it is critical to be able to evidence impact, demonstrating benefit from their research (e.g. behaviour change, competitive advantage in business, attracting foreign investment, and new or changed policy); without evidence it is of very limited use to them for appraisals. In the UK, impact is being driven into the appraisal structure by the government funding councils' inclusion of impact case studies in their assessment of research excellence (REF), and whilst an administrative burden this is a key mechanism used to encourage effective collaboration.
All funding proposals to the UK Research Councils require the creation of an impact summary describing who will benefit from the research and pathways to impact describing the approach that will be taken to deliver these impacts. Although traditionally weighted significantly lower than scientific excellence, one recent large funding scheme (the Industrial Strategy Challenge Fund of GBP 4.7 billion) weights impact only slightly less than excellence, and in another (the Global Challenges Research Fund of GBP 1.5 billion) it is the main objective (UKRI, 2017, 2018a); thus, applications to the funds require credible, significant and far-reaching impact for proposals to be able to be funded. The importance of impact is also growing in the UK's 7-yearly appraisal of research across the higher education sector, now accounting for 25 % of institutions' scores and significantly affecting league table rankings and income (i.e. REF2021; http://www.ref.ac.uk/, last access: 7 January 2019).
Within the insurance sector, we propose that types of impact and supporting evidence might include the following, although which of these is most important varies by a practitioner's role (e.g. broker, research manager or model developer). The list draws upon published material (Reed, 2018, chap. 22) and the experience of insurance sector amongst the co-authors.
-
Cost savings (e.g. saving on reinsurance), increased profit or an increase in turnover (e.g. by better pricing) where the research made a significant contribution to decision-making and operational utility (e.g. data or tools) that led to benefit. Economic benefits such as these may be evidenced via the following:
- a.
financial records (these can be clearly marked for the eyes of reviewers only and redacted for any public record)
- b.
reports in the mainstream media or business publications (e.g. Insurance Times), ideally stating the change or difference that has been made and linking this to the research
- c.
a testimonial letter describing the nature of the benefit in the risk practitioner's own words and how it arose from work with the researcher.
- a.
-
Improved strategic decision-making. This may include entering a new partnership or geographic region based on evidence from the research. Decision-making impacts like these may be captured in strategic documents and agreements; citing the published research in these documents makes it easier for researchers to claim impact. Otherwise, testimonials are widely used to evidence this sort of impact.
-
Capacity-building impacts. This may include new skills or business capabilities generated via internal training courses by researchers, based (at least partly) on their research. Evidence for this could include the amount of training conducted, feedback from participants, ideally indicating the effect the training has on their work, or any publicly available white paper, policy document, professional newsletter or blog stating the advantage gained through the research.
-
Understanding or awareness impacts. This includes uncovering the scale or urgency of a problem, perhaps of a peril (e.g. clustering of extra-tropical cyclones; e.g. Vitolo et al., 2009). There may be no solution to the problem at present, such as for flood–wind interdependency (e.g. Hillier et al., 2015; De Luca et al., 2017), but awareness may have an impact in itself, and in time lead to further impacts. Evidence could relate to recognition in blogs or business awards (e.g. Lloyd's Science of Risk Prize).
-
Reputational enhancement differentiating a company from its competitors. This is non-trivial to evidence, even internally within a business, so testimonials may be the only way to evidence this sort of impact.
-
Improved operational utility. Operational utility could have been improved where research has provided better inputs (e.g. data, methods or theories) with which to build better models or more robust views of risk. Evidence for impacts like these that are also pathways (e.g. to cost savings) may be captured via mentions or citations in policy documents or technical documentation.
6.2.3 Career
Tenure with its guaranteed job security (Adams, 2006) was legally abolished in 1988 by the UK government (Enders, 2015; Legislation, 1988). This opens up university research scientists to a much greater steer by appraisals (e.g. Costa and Olivera, 2012; Su and Baird, 2017) and via promotion criteria that are the universities' distillations of institutional and external policy expectations (Sect. 5.3).
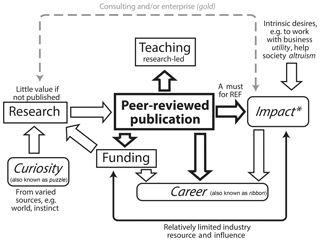
Figure 4Summary of relationships between academics' motivations, listed in Sect. 6.2 and 6.2.2 (italics), and main headings into which their main duties and appraisal criteria fall (Tables 1 and 2). Bolder, wider and blacker arrows indicate stronger relationships; in short, publication remains paramount (see main text). Of the four main motivations, gold is not prominent, only because this work focuses on the other three (i.e. impact, curiosity and career). Terminology of Lam (2011) for motivations is given in brackets. This conceptual model builds on previous work by integrating motivations with duties and by introducing impact as a motivation and the types of intrinsic desire behind it. The asterisk indicates that impact is both a requirement and a motivation.
A main finding here (i.e. Sect. 5) is that national-level policies to incentivise impact (i.e. REF and funding; Sect. 6.2.2) have now entered into the everyday consciousness of UK academics, with pervasive pressure to engage in impact-related work from institutions and funders; whilst enterprise or impact have propagated to be main headings in only three of the 10 institutions considered, all promotion documents contain criteria relating to enterprise or impact. In response, many academics now pursue impact to align with institutional requirements. Notably however, in terms of time allocation and duties, impact-related work is one task amongst many (i.e. Table 1, Fig. 3) and is likely only considered of value if it generates income to fund future research or is suitable for a REF impact case study. Evidentially, in practice, it also remains subservient in importance to research and teaching, thus it is wise and perhaps critical for work with business to facilitate or inspire better curiosity-led research (see Sect. 6.2.1). Lastly, to complete the contextual picture, it is necessary to understand that only a minority of UK academics are required to be heavily involved in KE (e.g. Reed, 2018); with ∼1 REF case study per 10 academics employed by a university department, required involvement is roughly 20 %–30 % of researchers.
Based upon the data in Sect. 5, Fig. 4 presents a new simplified model of the task facing an early- to mid-career university-based environmental scientist on the teaching and research pathway most commonly available. Firstly, it develops on existing models in that it integrates literature on motivation (D'Este and Perkmann, 2011; Freitas and Verspagen, 2017; Lam, 2011) and evaluation (Cadez et al., 2017; Grendon, 2008; Harland and Wald, 2018; Moya et al., 2015), building upon a view based around a teaching–research dipole and older (i.e. pre-impact emphasis in UK) ideas (Hughes et al., 2008). This integration sheds light on why these motivations arise. Secondly, it is modified to include work with business (i.e. impact) and the two intrinsic motivations behind this identified in this study (utility and altruism; Sect. 6.2). Given that neither the government (i.e. UKRI) view nor REF vary notably between academic sectors (e.g. environmental science, social science and engineering), we propose that the model is widely applicable. The model's utility here is that it is a framework that gives insight into why particular modes of engagement proposed later (i.e. Sect. 6.3) might be successful instead of languishing incomplete.
Publishing novel science in peer-reviewed journals is an academic's overriding imperative (e.g. Hattie and Marsh, 1996), followed by winning funding to facilitate publications (i.e. by funding a post-doctoral researcher). Publications (bold box and arrows on Fig. 4) are the critical appraisal measure, as they demonstrate success in research and underpin teaching, impact, career (i.e. promotion, mobility or simply retaining a job), reputation and future funding bids (Sect. 6.1.4). Funding, or more generally, resources (i.e. PhD student time, post-doctoral researchers or income), is an important appraisal criterion, as it indicates reputation and the ability to undertake research but is significant to an academic as a career measure in its own right. Research itself is marked in a lighter font (Fig. 4), as it is ascribed little value until published. Impact is light, as its influence upon an academic's assessment (i.e. career) is still relatively limited, although research and impact promotion pathways are now possible at some universities. REF and TEF, and soon KEF (i.e. Knowledge Exchange Framework; https://re.ukri.org/knowledge-exchange/knowledge-exchange-framework/, last access: 7 January 2019), assess UK universities in research, teaching and KE. So impact may gain weight as KEF is brought in, although it is unlikely to exceed REF or TEF in importance. However, it is key to note that all three assessments are ultimately underpinned by peer-reviewed publications of original work. With REF, this is direct, TEF is driven by research-led teaching, and KE and impact are required to be tied back to published outputs. Thus, even fully buying out an academic to do work for impact with substantive funding (e.g. a 2-year KE Fellowship) does not currently reduce the model's applicability for a scientist wishing to remain in academia.
The entrepreneurial route directly between research and impact (Fig. 4) is indicated with a dashed line, as it is relatively uncommon (Lam, 2011), and the arrow from impact to funding is thin to reflect the current relative influence it has on the magnitude of resources. Teaching and leadership and administration are obligatory but will not get a scientist shortlisted for a job, so they are not focussed on in Fig. 4, even if they are required to actually obtain the job. Motivations detailed in Sect. 6.2 are in italic typeface, whilst the main headings that duties and appraisal criteria fall into are in normal typeface.
The important thing for a risk practitioner to recognise is that if an academic already under substantial time pressure (Sect. 6.1.1) wishes to engage with business, their only solution is to be effective, efficient and prioritise carefully to select what they will not do, and usually the only flexible element is their own research (e.g. see Bothwell, 2018). Thus, such real-world impact must inspire curiosity and provide some way of better doing new science (e.g. ideas, access to novel data, resources or a PhD student), at least in the longer-term element of an academic career (see the dynamical model including “use inspired basic research” from Stokes, 1997, for theoretical context; e.g. Cantisani, 2006, Figs. 2 and 3). If this feedback exists, the academic might be able to find up to about half a day per week, but such a large time sacrifice would need substantial incentives (Sect. 6.1.1).
6.3 Practical hints and tips to build collaboration
The objective of collaboration is to translate business-relevant questions (Dixon et al., 2017; e.g. Lighthill Risk Network, 2016) into research questions that are precise enough for scientists to be able to answer and intriguing and novel enough for scientists to want to prioritise answering them, then deliver outputs of benefit to all. A vital element of successful collaboration is a long-term trusting relationship (e.g. Dowling, 2015). Despite such a relationship being an ongoing and time-intensive venture to construct, it is critical in overcoming a number of barriers such as IP (Sect. 6.1.3), language (e.g. Fish and Saratsi, 2015; Jordan and Huitema, 2014; Scott et al., 2018), differing working and problem solving cultures (e.g. Amabile et al., 2001), or simply contextual knowledge and credibility (e.g. Hughes et al., 2008); just as an academic's motivations and skills may not be transparent to the wider world, academics do not instinctively have an intimate knowledge of any insurer's technical approach, recent initiative or strategic internal drivers. Of particular relevance is the difficultly that novelty (i.e. for publications) and immediate industrial implementation (e.g. in operational risk models) are not directly compatible in the same task, although steps that will mainly benefit one party or both can be balanced over time throughout a co-designed project.
Many conceptual models of the knowledge exchange process exist (e.g. Jacobson et al., 2005; Ward et al., 2009). No particular one is assumed, although cyclic, iterative and two-way elements are recommended.
Below we propose two illustrative, non-exhaustive lists of pragmatic suggestions for time-efficient activities that have reportable and mutually beneficial outputs in order to build the long-term trusting relationship needed for collaboration between an academic and a risk practitioner. Each suggestion includes an explanation of why the activity has benefit or utility to justify time spent on it, mapped back to appraisal criteria (Table 2) or an impact typology (Sect. 6.2.2). Obvious relationship-building and maintenance activity is assumed, e.g. short chats over a coffee, telephone calls, passing on interesting items (e.g. an article or newspaper clipping) and mutual tolerance of unavoidable busy periods (e.g. Sect. 5.2).
The following list details ways in which risk practitioners can support an academic partner, including a brief commentary on how and why benefits emerge. Square brackets, e.g. [2], indicate mapping the criteria by which academics are assessed in Table 2. Suggestions are not ordered, as their relative utility will be case specific.
-
Write a letter of support and engagement on a research grant application [4]. This is a useful relationship-building measure and will be best when projects are co-designed. They are required for UK funding applications, but if sought at the last minute after limited discussion, it often remains unclear why the insurer should prioritise this action (i.e. what the benefits of the work might be), potentially leading to mutual frustration. In contrast, a timely discussion leading to a letter of support which indicates the scale of potential impact of the research to the industrial partner, and detailed pathways to it (e.g. specific committees, regulatory compliance requirements or internal initiatives), would significantly strengthen a research grant application.
-
Offer a place on an advisory panel, committee or a similar position [8, 9, and potentially 3 and 4]. Even with a non-disclosure agreement in place, this could be useful to an academic on many fronts; a role title enhances their CV and/or annual appraisal, and even a small remuneration (e.g. ∼ GBP 1000 in a year) looks good as an income source from business. Furthermore, as such money is undesignated, it is ideal for buying a little of a research assistant's time (e.g. a PhD student) to do a pilot study; these greatly help when writing funding bids for substantive money, ultimately leading to publications. If the academic were also able to say the advice stemmed from a published paper, this would be evidence of impact outside academia, particularly with a supporting statement (e.g. “advice on earthquake clustering was provided, drawing on X's recent publications, contributing to an evaluation of two catastrophe models of natural hazard risk”). On both sides, it is another chance to meet, talk and build a relationship.
-
Request a few (e.g. 1–3) days of consultancy [8, 9, and potentially 3 and 4]. This may include commenting on a catastrophe (i.e. natural hazard risk) model's documentation. The money and impact benefits are as described above. This is likely a loss leader for the academic but is something useful that is safe, will happen, and is measurable and reportable. In some ways, this mirrors the literature review that they can do for you when written into a project grant (see below list of ways research scientists might support risk practitioners).
-
Provide access to data [9 and first step to 3 and 4]. This is to allow a novel insight into a scientific problem, and could be very useful for the academic and pique their curiosity but will likely need significant funding to fully implement. This being said, a data-driven pilot study could be of immediate use to the risk practitioner and also give a strong core to an academic's bid for funding.
-
Offer grant funding for “innovation” or highly applied work [4, 8 and 9]. This may either be direct (e.g. AXA Research Fund) or via government funding that requires an industrial lead (e.g. Industrial Strategy Challenge Fund, Innovate UK). This is good for an appraisal's funding metric and can provide a means of moving towards evidence of impact outside academia, but it is unlikely to produce highly novel, inventive and cutting-edge scientific research. So this is useful, either in the relatively short-term (1–3 years) or in parallel with blue-skies funding, but is insufficient alone in the longer-term period for the scientist.
-
Fund blue-skies research [3 and 4]. This is an ideal scenario for many academics and is done by a number of organisations (e.g. Willis Towers Watson or AXA), but the sums are relatively large; the full economic cost in the UK for a post-doctoral research assistant for 2–3 years needed to make a post attractive to a good candidate is ∼ GBP 200 000. External (e.g. UKRI) funding lowers costs but also reduces control over the topic, scope of the work (i.e. funder's requirements), and whether work will be funded or not.
-
Collect evidence of impact [9]. Impact is diverse, and evidence is not onerous to obtain (see Sect. 6.2.2). Creating an impact case study for the REF exercise can win internal (i.e. university) investment in the form of time or money, freeing the academic to pursue further research or develop this strand of impact.
-
Co-design a research project for external funding [3, 4 and 9]. Impact designed at a project's inception (e.g. Reed, 2018) can inspire world-leading science and publications in the highest-impact journals, with ideas and inspiration possible from all parties. However, preparatory conversations over time are needed to ensure there will be novel insights into the underpinning physical processes at work as well as real-world impacts. Early in a collaboration this may take substantial time (e.g. 6–12 months). It is important to note that the impact and novel science do not need to come at exactly the same time or from precisely the same task, i.e. distinct outcomes particular to both risk the practitioner (see below) and academic (e.g. publications) should be separately identified. Ultimately, even if both scientist and practitioner are time-limited, co-design in an established relationship can be efficient, i.e. a route to better research that is also faster (e.g. Amabile et al., 2001). UKRI-funded options range from a PhD studentship (e.g. via CASE awards), KE Fellowships or innovation placements to NERC standard grants or Global Challenges funding calls (see Sect. 6.2.2.).
-
Ask them to provide training [9]. If a clear fit exists, paying an academic to provide in-house training is a good way to get to know them, which the academic can justify in the same way as consultancy.
-
Provide access to training, expertise (e.g. actuaries) or networks. This is primarily a mechanism to maintain contact and alignment, since academics are typically proficient at obtaining these already.
Although apparently a counterpoint to the main theme of this article, aimed at risk practitioners, an illustrative list of actions a university scientist may take to support their risk practitioner is given below; it may assist practitioners new to the role of collaboration with academics or as an aid to give to an academic new to collaborating with insurers.
In this spirit, it is worth giving a precis of motivations within this industrial sector. As individuals, it is notable that a practitioner's motives are mixed, with curiosity (i.e. the puzzle) and family as common drivers instead of just the gold (see Sect. 6.2; Lam, 2011). Whilst insurers ultimately require increased profitability, and approaches to quantify this to create a business case for collaboration are mixed and varied, three main routes exist: training, operational utility (e.g. data and tools) or reputational enhancement. The latter works by differentiating the company from its competitors (i.e. more accurate risk assessment through better science), providing arguments for retaining existing clients and opening doors to new clients that sales teams can follow up on. In (re)insurance this can be more important than harvesting and protecting IP generated in collaborations.
The following list is of ways research scientists might provide support to their risk practitioner partner. These are mapped to the typology of impact (i.e. practitioner benefit) in Sect. 6.2.2 using square brackets, e.g. [2], and include a brief commentary on how and why benefits emerge. Suggestions are not ranked, as utility will be case specific.
-
Undertake a literature review [4]. This could be a comprehensive review of what is known about risks in an emerging peril-region, perhaps in Africa. This is a safe (i.e. low risk), early-stage deliverable if included into funding bids. It will appear the least like a burden to the academic if the subject is novel (i.e. publishable) and a likely impact (e.g. pending strategic decision) has been identified. It is time efficient for the practitioner.
-
Deliver new research-based science [1]. This could be in the form of concepts or theories that can be implemented by the practitioner for operational advantage ahead of competitors, e.g. by engaging with the scientist in a co-designed project as the work progresses. Feed-in could be by modifying a company's “own view of risk”, or by some adaption to their natural hazard risk process and model (e.g. catastrophe modelling). When exploring ideas or methodological improvements at the cutting edge (i.e. higher risk), collaboration can be a low-cost alternative for a practitioner, since if sufficient novelty exists, a substantial fraction of the cost might be supportable through public funding.
-
Develop a spreadsheet-based decision support tool [1]. Although this is too basic for most (re)insurance users, it may be appropriate for some of their clients.
-
Provide training sessions [3]. See list above.
-
Create a software tool (e.g. in R-shiny) associated with a statistical model developed during research [1, 2, 4]. This should be associated with a statistical model developed during research [1, 2, 4]. Such accessible, interactive visualisations can raise awareness amongst internal management or external clients of saleable new functionality or product opportunities. Some practitioners encourage dissemination as supplementary material to a journal article.
-
Develop a simplistic exposure-based natural hazard risk model, for example, a catastrophe model. This should be of just sufficient complexity to illustrate a particular scientific insight (e.g. Royse et al., 2014). The rationale is similar to the software tool.
-
Provide expert advice [2, 4, 5]. This could be to an internal and/or external committee or decision-making group, perhaps as ad hoc input on the latest science. This is time-effective for the practitioner and aligns with the academic's interests (see above).
-
Contribute to business forums and conferences, for example, of RMS, AIR, Aon and Oasis, on co-designed work [4 and 5]. This should highlight a practitioner's engagement with the latest science.
-
Produce footprints for a catalogue of historical events [1]. This is perhaps the easiest aspect of a catastrophe model for environmental scientists to contribute to. Other elements (e.g. vulnerability functions or stochastic event sets) either require sensitive data (i.e. claims) or being fully benchmarked against business standards before they could be operationalised.
-
Invite the practitioner to give a guest lecture (for example, to undergraduates) seminar or training [5]. This is a potentially enjoyable experience, an opportunity to discuss collaboration possibilities and provides contact with students (e.g. PhD) who may apply for jobs with the company in future.
These lists are not, and do not attempt to be, exhaustive (e.g. short placements of 1 week). The key is open, honest and continual interaction based on an appreciation of motives, which may help to bridge frustrating gaps. Currently, provision of hazard footprints illustrates this; business asserts a need for accessibility (e.g. on OasisHub) in a business data format, yet it is difficult for a researcher to prioritise doing this on only the speculation that impact may happen. With concrete and specific plans for creating and collecting detailed evidence of impact in place with the insurer, the academic may readily see the value in sacrificing research to do the work. Alternatively, a brief session to advise a consultant paid to undertake the work of making the footprints easily usable may be all that can be justified (e.g. see Moulin, 2018:47).
More widely we note workshops (e.g. Dixon et al., 2017), brokerage events (e.g. for funding calls), industrially funded initiatives (e.g. the Willis Research Network or JBA Trust), the use of distinct and separate middle people as translators or facilitators (e.g. consultants or NERC's KE Fellows), and institutional-scale university responses (e.g. “business liaison officers”). These broader and/or institutional approaches might sit alongside and support engagement established and maintained at the individual level, but they do not alter the findings of this work. Ultimately, all the approaches must be consistent with the motives of the individual academics whose “core business” remains researching environmental science. We also note that longer (i.e. 1 week) placements for either party (i.e. academic or practitioner) may also be valuable but entail an extended absence from the employer and work environment (e.g. as described in Sect. 5) and are thus out of scope of these collaboration-building recommendations, as they are more substantial and costly undertakings (i.e. requiring buying out an academic's time) and will likely come later in a relationship. This similarly applies to large UKRI-funded initiatives (e.g. large grants, fellowships and Knowledge Transfer Partnerships).
6.3.1 Summary of pragmatic ways to nurture collaboration
A trusting long-term relationship is vital, but it has to start somewhere. In brief, a mixture of short-term steps (<1 year) to initiate a relationship, building toward longer-term and more substantive targets and outputs (1–5 years), is suggested. The ideal is mutually beneficial at each stage; even if consultancy and giving advice are not what is ultimately critical to a university-based scientist (i.e. subject-leading publications), most are patient in developing towards this (e.g. industrial relationship and funding bids). The advice may act as a template or basis to design guides in other industrial sectors.
Since (re)insurance businesses are typically multinational, and academic motivations (e.g. funding or publications) are similar internationally, the advice on initiating collaborations is not specific to the UK, as it only assumes a pre-existing motivation towards impact. Internationally, Australia's Excellence in Research for Australia (ERA) exercise in 2018 (http://www.arc.gov.au/era-2018, last access: 7 January 2019) is now partnered by an Engagement and Impact Assessment (EI; http://www.arc.gov.au/engagement-and-impact-assessment, last access: 7 January 2019), providing a well-documented example of the growing weight given to impact in a number of economically developed countries. In terms of a conceptual model of the framework in which academics work (Sect. 6.2.3), all indications are that the UK is usefully representative. The CAP survey of 100 academics (2004 to 2012; Teichler et al., 2013) describes a self-reported mean workload of ∼48 h per week across 18 countries, remarkably consistent with the 45–50 h per week in the UK and in line with Australia, which is about the middle of the spectrum (Fig. 17 of Coates et al., 2009). With 65 %–89 % of university scientists, depending upon country, having a prime interest in research rather than teaching (Abreu et al., 2009; Cavalli and Moscati, 2010), the UK is also typical in this regard. The CAP survey (Teichler et al., 2013) also reported teaching as 38 %–46 % of work hours, and ratios of research to teaching in a range of countries (e.g. UK, Finland and Portugal) are near parity. Furthermore, the literatures on motivation (D'Este and Perkmann, 2011; Freitas and Verspagen, 2017; Lam, 2011) and evaluation (Cadez et al., 2017; Grendon, 2008; Harland and Wald, 2018; Moya et al., 2015) from which our model developed are international (i.e. Netherlands, New Zealand, North America, Slovenia, Spain and UK). As such, we suggest that the conceptual model proposed in Sect. 6.2.3 has global applicability and transferability or that it is at least a suitable basis for future discussion; a key variant will be the strength of the impact–career link. Our conceptual model's applicability may be limited as academic systems vary by country (Cavalli and Moscati, 2010; Coates et al., 2009). However, in general, tenure with its guaranteed job security (Adams, 2006) has declined (e.g. in the USA), or been eliminated entirely (e.g. UK; Finkelstein, 2010; Huisman et al., 2002). In Germany a job-for-life system remains, but since the 1980s in the UK and Netherlands, university staff are employed by their institution and not the state (Enders, 2015). This opens up many international research scientists to a much greater steer by appraisals (e.g. Costa and Olivera, 2012; Su and Baird, 2017) and via promotion criteria.
The need to juggle research, teaching and leadership and administration demands has been reported for Australian institutions (Coates et al., 2009; Lazarsfeld-Jensen and Morgan, 2009). Interestingly, Australian professors work 52.2 h per week, more than SLs at 46.4 h per week, with the difference made up by research. In other words, with other things (i.e. teaching or leadership and administration) non-optional, a stronger staff profile appears to be created by working more hours to do research (Coates et al., 2009), although this is likely detrimental to academics and institutions (Lazarsfeld-Jensen and Morgan, 2009), e.g. 100 % of 91 Australian academics reported working weekends, 43 % of these in the 37–48 weekend per year bracket. So Australia is perhaps an even more extreme example than the UK. A number of co-authors have worked as academics in other countries (e.g. Germany, South Africa and USA), and all work closely and openly with international collaborators. Their experience supports the view that academic behaviours are similar outside the UK, except that institutional pressure for impact is usually lower; however, such pressures are developing (e.g. in Australia and Germany). So, this paper may be of interest in understanding the trajectory of the academic environment in a number of nations.
This paper in itself provides an illustration of the practicalities involved in creating benefit for an academic via evidence-based impact; even if you do change behaviour after reading it, no feedback to items of concrete value to the academic co-authors (i.e. appraisal and REF impact case study) is possible without evidence. And how will the university-based scientist find out if they are not told? Thus, dear reader, if this paper has had any influence on you, it would be valuable for us to hear from you, and we would be grateful for any comments. Even a one or two line e-mail to the authors (j.hillier@lboro.ac.uk) noting any changes or potential plans to change (e.g. “I might now consider using an academic for internal training”) would class as evidence. Readers are kindly reminded that even raising awareness is classed as impact (Reed, 2018; Sect. 6.2.2).
Based on an innovative mixed-method approach, and using an original empirical dataset, the main findings of this work are about the nature of the challenge posed by the heavily time-constrained culture of today's universities. Specifically, we assess exactly how individual scientist's workload (i.e. specified tasks) and incentive structures (i.e. assessment criteria) may act as a key barrier to university–business collaboration. Two initial conclusions are as follows.
-
Time is limited. Amid a raft of 20–50 key duties, typical full-time university-based scientists may be able to free up to 0.5 days per week for work with practitioners (i.e. impact activities) in sectors such as (re)insurance.
-
Pragmatic strategies are needed. Given the time limitations on both parties (e.g. academics and practitioners), it is necessary to establish coping strategies and determine pragmatic steps to secure initial traction and to build a relationship.
Insights are also obtained into why academics' motivations arise, what governs the relative dominance of these motivations and how exactly time constraints manifest themselves in academics' behaviours in the presence of impact requirements. Importantly, tension is shown to typically exist between exciting curiosity-driven opportunities in university–business collaboration and workload. Thus, to justify the time to collaborate with business, the work must inspire curiosity and facilitate future cutting-edge and world-class science in order to mitigate the conflict with an academic's overriding imperative to publish. It must also provide evidence of real-world changes, and ideally other reportable outcomes (e.g. official status as an insurer's advisor), to feed back into the scientist's performance appraisals. It is therefore important to formulate questions that are precise enough for the scientist to be able to answer and are intriguing and novel enough for the scientist to want to prioritise answering them.
New understanding is encapsulated in an improved conceptual model (Fig. 4) of the inter-relationships between day-to-day key duties, performance assessment, and longer-term motivations and aspirations in an academic job. The main developments of this model are
-
Including impact. Impact is now included in the tensioned relationship between elements of an academic job, building on models that only considered a teaching–research dipole.
-
Identifying new impact-related motivations. In addition to career (also known as ribbon; Lam, 2011), academics' desire to work with business, be useful (i.e. utility) and to aid society (i.e. altruism) as intrinsic drivers for undertaking impact-related work.
-
Including deeper understanding of motivations. Light is shed on why academic motivations arise and the relative magnitude of their influence on academic behaviour, using a novel source of data and by integrating separate literatures on academic motivation and evaluation.
Finally, we have shown that a variety of pragmatic short-term (<1 year) steps can be proposed for à la carte use to initiate and nurture a relationship between academia and business. Based upon the understanding gained from the analysis, explanation is provided as to how these mitigate the disincentives within today's academic environment, align with business needs and contain the potential for mutual benefit at each stage. These are designed to build toward longer-term and more substantive targets and outputs (1–5 years), with detail specific to environmental scientists and risk practitioners provided.
More widely, the discussion provides a window into the motives of university-based research scientists that, in addition to practitioners, will be highly relevant to a number of academic colleagues, university administrators and policymakers. The model and recommendations are derived from UK data but are likely of interest internationally (e.g. Australia and Europe), although this is a question for future study.
Metadata (e.g. web links) for the 20 sets of documents for UK job specifications and promotion criteria are given in the Supplement but are not supplied for copyright reasons. Personal data for the KEN participants are not provided or retained, but derived data are given within the paper (i.e. Table 1) or in the Supplement, i.e. for the word clouds (Figs. 1, 2).
The supplement related to this article is available online at: https://doi.org/10.5194/gc-2-1-2019-supplement.
JH designed the study, led data collection and undertook initial drafting of the paper. All authors contributed observational, experience-based data about academics' behaviour and/or (re)insurance-based practice, and contributed to the writing of the paper.
The authors declare that they have no conflict of interest.
John K. Hillier was funded by NERC grant NE/R003297/1. We thank the attendees
at NERC's KEN workshop in June 2018 for participating.
Mark Reed's
comments much improved an early version of this paper, as did
James Esson's comments on a later version. We are grateful to Richard Westaway and
Anson Mackay for their thoughtful and constructive reviews of this work. All
views are those of individuals and not the organisation(s) they
represent.
Edited by: Jon
Tennant
Reviewed by: Anson Mackay and Richard Westaway
Abreu, M., Grinevich, V., Hughes, A., and Kitson, M.: Knowledge Exchange between academics and the business, public and third sectors, available at: https://www.jbs.cam.ac.uk/fileadmin/user_upload/centre-for-business-research/downloads/special-reports/specialreport-knowledgeexchangeacademics.pdf (last access: 7 January 2019), 2009.
Adams, M. L.: The quest for tenure: Job security and academic freedom, Cathol. Univers. Law Rev., 5, 67–97, 2006.
Amabile, T., Patterson, M., Mueller, C., Wojcik, J., Odomirok, P. W., Marsh, M., and Kramor, S. J.: Academic practitioner collaboration in management research: a case of cross-profession collaboration, Acad. Manage. J., 44, 418–431, 2001.
Amsterdamska, O.: Book Review: Surely You Are Joking, Monsieur Latour!, Sci. Technol. Hum. Val., 15, 495–504, https://doi.org/10.1177/016224399001500407, 1990.
Arnold, I. J. M.: Course level and the relationship between research productivity and teaching effectiveness, J. Econ. Educ., 39, 307–321, 2008.
Bercovitz, J. and Feldman, M.: Entrepreneurial universities and technology transfer: A conceptual framework for understanding knowledge-based economic development, J. Technol. Transfer, 31, 175–188, 2006.
Bostrom, M., Lidskog, R., and Uggla, Y.: A reflexive look at reflexivity in environmenal sociology, Environ. Sociol., 3, 6–16, 2017.
Bothwell, E.: Work-life balance survey 2018: long hours take their toll on academics, Times High, Eduction, available at: https://www.timeshighereducation.com/features/work-life-balance-survey-2018-long-hours-take-their-toll-academics, last access: 6 July 2018.
Broch, L., Rasilla del Moral, I., Ajoha, R., Afonso, A., Ianni, A., Walker, N., and Muttarak, R.: United Kingdom, Academic Career Structure, available at: https://www.eui.eu/ProgrammesAndFellowships/AcademicCareersObservatory/AcademicCareersbyCountry/UnitedKingdom (last access: 20 May 2018), 2017.
Cadez, S., Dimoviski, V., and Groff, M. Z.: Research, teaching and performance evaluation in academia: the salience of quality, Stud. High. Educ., 42, 1455–1473, https://doi.org/10.1080/03075079.2015.1104659, 2017.
Cantisani, A.: Technological innovation processes revisited, Technovation, 26, 1294–1301, 2006.
Carayol, N.: Objectives, agreements and matching in science-industry collaborations: Reassembling the pieces of the puzzle, Res. Policy, 32, 887–908, 2003.
Cavalli, A. and Moscati, R.: Academic Systems and Professional Conditions in Five European Countries, Eur. Rev., 18, S35–S53, 2010.
Coates, H., Dobson, I., Edwards, D., Friedman, T., Goedegebuure, L., and Meek, L.: The attractiveness of the Australian academic profession: a comparative analysis, Melbourne, Vic. LH Martin Institute, Univ. Melb. Aust. Counc. Educ. Res. Educ. Policy Institute, 39 pp., available at: http://hdl.handle.net/11343/28921 (last access: 25 May 2018), 2009.
Collette, A., Leith, N., Daniel, V., Bellone, E., and Nolan, D. S.: Using Mesoscale Simulations to Train Statistical Models of Tropical Cyclone Intensity over Land, Mon. Weather Rev., 138, 2058–2071, https://doi.org/10.1175/2010MWR3079.1, 2010.
Costa, B. E. and Olivera, M. D.: A multicriteria decision analysis model for faculty evaluation, Omega, 40, 424–436, https://doi.org/10.1016/j.omega.2011.08.006, 2012.
De Luca, P., Hillier, J. K., Wilby, R. L., Quinn, N. W., and Harrigan, S.: Extreme multi-basin flooding linked with extra-tropical cyclones, Environ. Res. Lett., 12, 114009, https://doi.org/10.1088/1748-9326/aa868e, 2017.
Denscombe, M.: The Good Research Guide: For Small-Scale Social Research Projects, 4th Edn., Open University Press, 2010.
D'Este, P. and Patel, P.: University-industry linkages in the UK: What are the factors determining the variety of interactions with industry?, Res. Policy, 36, 1295–1313, 2007.
D'Este, P. and Perkmann, M.: Why do academics engage with industry? The entrepreneurial university and individual motivations, J. Technol. Transfer, 36, 316–339, 2011.
Dixon, R., Souch, C., and Whitaker, D.: European windstorm: Needs of the insurance industry, available at: http://www.stormworkshops.org/workshop2017.html (last access: 7 January 2019), 21–23 June 2017, Reading, UK, 2017.
Dowling, D. A.: The Dowling Review of Business-University Research Collaborations, available at: https://www.gov.uk/bis (last access: 7 January 2019), 2015.
Drexl, J.: Designing competitive markets for industrial data – between propertisation and access, Max Planck Inst. Innov. Compet. Res. Pap. No. 16-13, 2016.
Dutta, S., Lanvin, B., and Wunsch-Vincent, S.: The Global Innovation Index 2016: Winning with Global Innovation, available at: https://www.globalinnovationindex.org/userfiles/file/reportpdf/gii-full-report-2016-v1.pdf (last access: 7 January 2019), 2017.
Enders, J.: Explainer: how Europe does academic tenure, Conversat, available at: https://theconversation.com/explainer-how-europe-does-academic-tenure-43362 (last access: 28 May 2018), 2015.
Etzkowitz, H.: Research groups as “quasi-firms”: The invention of the entrepreneurial university, Res. Policy, 32, 109–121, 2003.
Evans, D.: Academics do want to engage with business, but need more support, Conversat., available at: http://theconversation.com/http://theconversation.com/ (last access: 7 January 2019), 2016.
Feller, I.: Universities as engines of R&D-based economic growth: They think they can, Res. Policy, 19, 335–348, 1990.
Finkelstein, M. J.: Diversification in the Academic Workforce: The Case of the US and Implications for Europe, Eur. Rev., 18, S141–S156, 2010.
Fish, R. and Saratsi, E.: Naturally Speaking… A Public Dialogue on the UK National Ecosystem Assessment, Final Report, CRPR, University of Exeter, Exeter, UK, 2015.
Florida, R. and Cohen, W. M.: Engine or infrastructure? The university role in economic development, in: Industrializing knowledge: University-industry links in Japan and the United States, 589–610, MIT Press, Cambridge, 1999.
Freitas, I. M. B. and Verspagen, B.: The motivations, institutions and organization of university-industry collaborations in the Netherlands, J. Evol. Econ., 27, 379–412, 2017.
Gendron, Y.: Constituting the academic performer, Eur. Account. Rev., 17, 97–127, 2008.
Goff, F.: NERC Impact Report, available at: https://nerc.ukri.org/about/perform/reporting/reports/impactreport2015/ (last access: 7 January 2019), Natural Environment Research Council, 32, 2015.
Grendon, Y.: Constituting the Academic Performer: The Spectre of Superficiality and Stagnation in Academia, Eur. Account. Rev., 17, 273–278, 2008.
Grimple, C. and Fier, H.: Informal university technology transfer: A comparison between the United States and Germany, J. Technol. Transfer, 35, 637–650, https://doi.org/10.1007/s10961-009-9140-4, 2010.
Harland, T. and Wald, N.: Vanilla teaching as a rational choice: the impact of research and compliance on teacher development, Teach. High. Educ., 23, 419–434, https://doi.org/10.1080/13562517.2017.1395408, 2018.
Hattie, J. and Marsh, H. W.: The Relationship Between Research and Teaching: A Meta-Analysis, Rev. Educ. Res., 66, 507–542, 1996.
HEFCE: REF 2014: Key facts, available at: http://www.ref.ac.uk/2014/pubs/keyfacts/ (last access: 28 May 2018), 2015.
HESA: Higher Eduction Staff Statistics, available at: https://www.hesa.ac.uk/data-and-analysis/staff (last access: 7 January 2019), 2018.
Hillier, J. K., Macdonald, N., Leckebusch, G. C., and Stavrinides, A.: Interactions between apparently primary weather-driven hazards and their cost, Environ. Res. Lett., 10, 104003, https://doi.org/10.1088/1748-9326/10/10/104003, 2015.
Hillier, J. K., Foote, M., Tsanakas, A., Wardman, J., Mitchell-Wallace, K., Hughes, R, Dixon, R., Simeonova, B., and Brown, C.: Investing in science for natural hazards insurance, https://doi.org/10.17028/rd.lboro.c.4322666, 2018.
Hughes, T., O'Regan, N., and Wornham, D.: The credibility issue: closing the academics/practitioner gap, Strat. Chang., 17, 215–233, 2008.
Huisman, J., de Weert, E., and Bartelse, J.: Academic Careers from a European Perspective, J. High. Educ., 73, 141–160, 2002.
Jacobson, N., Butterill, D., and Goering, P.: Consulting as a strategy for knowledge transfer, Milbank Q., 83, 299–321, 2005.
Jordan, A. and Huitema, D.: Policy innovation in a changing climate: sources, patterns and effects, Global Environ. Chang., 29, 387–394, 2014.
KCC: 2017 Hurricane Season: Review and Analysis, KCC WHITE Pap., available at: http://www.karenclarkandco.com/news/publications/, last access: 16 July 2018.
Kemmis, S., McTaggart, R., and Nixon, R.: The action research planner: Doing critical participatory action research, Springer, 2013.
Lam, A.: What motivates academic scientists to engage in research commercialization: “Gold”, “ribbon” or “puzzle”?, Pol. Res., 40, 1354–1368, 2011.
Lambert, R.: Lambert review of university-business collaboration, final report, HM Treasury, London, UK, 2003.
Latour, B.: How to Follow Scientists and Engineers through Society, Harvard University Press, 1987.
Lazarsfeld-Jensen, A. and Morgan, K. A.: Overload: the role of work-volume escalation and micro-management of academic work patterns in loss of morale and collegiality at UWS: the way forward, 67 pp., available at: https://www.researchgate.net/publication/269279442_Overload_the_role_of_work-volume_escalation_and_micro-management_of_academic_work_patterns_in_loss_of_morale_and_collegiality_at_UWS_the_way_forward (last access: 28 May 2018), 2009.
Legislation: Education Reform Act 1988, 302 pp., available at: http://www.legislation.gov.uk/ukpga/1988/40/contents (last access: 25 May 2018), 1988.
Lighthill Risk Network: Key Research Themes & Challenges, available at: https://lighthillrisknetwork.org/research-priorities/ (last access: 28 May 2018), 2016.
Link, A. N., Siegel, D. S., and Bozeman, B.: An empirical analysis of the propensity of academics to engage in informal university technology transfer, Ind. Corp. Change, 16, 641–655, 2007.
McAreavey, R.: Getting Close to the Action: The Micro-Politics of Rural Development, Sociol. Ruralis, 46, 85–103, 2006.
McMillan Group: University Knowledge Exchange (KE) Framework: good practice in technology transfer, Rep. to UK High. Educ. Sect. HEFCE, available at: http://dera.ioe.ac.uk/27123/1/2016_ketech.pdf (last access: 9 July 2018), 2016.
Mitchell-Wallace, K., Jones, M., Hillier, J. K., and Foote, M.: Natural Catastrophe Risk Management and Modelling: A Practitioner's Guide, Wiley, Oxford, UK, 2017.
Morris, S., Pitt, R., and Manathunga, C.: Students' experiences of supervision in academic and industry settings: results of an Australian study, Assess. Eval. High. Educ., 37, 619–636, https://doi.org/10.1080/02602938.2011.557715, 2011.
Moulin, J.: Innovation-oriented cooperation of Research Infrastructures, ESFRI Scr., available at: http://www.esfri.eu/sites/default/files/u4/ESFRI_SCRIPTA_VOL3_INNO_single_page.pdf, last access: 9 July 2018.
Mowrey, D. C. and Nelson, R. R. (Eds.): Ivory tower and industrial innovation: University-industry technology before and after the Bayh-Doyle Act, Stanford University Press, Stanford, 2004.
Moya, S., Prior, D., and Rodriguez-Perez, G.: Performance-based Incentives and the Behaviour of Accounting Academics: Responding to Changes, Account. Educ., 24, 208–232, 2015.
Olsen, M.: Exploring the relationship between money attitudes and Maslow's hierarchy of needs, Int. J. Consum. Stud., 28, 83–92, 2004.
Owen-Smith, J.: From separate systems to a hybrid order: Accumlative advantage across public and private science at Research One universities, Res. Policy, 32, 1081–1104, 2003.
Perkmann, M. and Walsh, K.: University-industry relationships and open innovation: Towards a research agenda, Int. J. Manag. Rev., 9, 259–280, 2007.
Reed, M. S.: The research impact handbook, 2nd Edn., Fast Track Impact, Newcastle University, UK, 379 pp., 2018.
Rogers, E. M.: Diffusion of innovations, 5th Edn., Simon & Schuster, London, UK, 2003.
Royse, K., Hillier, J. K., Wang, L., Lee, T. F., O'Neil, J., Kingdon, A., and Hughes, A.: The application of componentised modelling techniques to catastrophe model generation, Environ. Modell. Softw., 61, 65–77, https://doi.org/10.1016/j.envsoft.2014.07.005, 2014.
Scott, A., Carter, C., Hardman, M., Grayson, N., and Slayney, T.: Mainstreaming ecosystem science in spatial planning practice: Exploiting a hybrid opportunity space, Land Use Policy, 70, 232–246, https://doi.org/10.1016/j.landusepol.2017.10.002, 2018.
Slaughter, S. and Leslie, L. L.: Academic capitalism: Politics, policies and the entrepreneurial university, John Hopkins University Press, Balimore, MD, 1997.
Stephan, P. E. and Levin, S. G.: Striking the Mother Lode in Science: the Importance of Age, Place and Time, Oxford University Press, Oxford, UK, 1992.
Stokes, D.: Pasteurs Quadrant: Basic Science and Technological Innovation, Brookings Institution Press, Washington, DC, USA, 1997.
Su, S. and Baird, K.: The association between performance appraisal systems, work-related attitudes and academic performance, Financ. Acc. Man., 33, 356–372, https://doi.org/10.1111/faam.12128, 2017.
Teichler, U., Arimoto, A., and Cummings, W. K.: The Changing Academic Profession – Major Findings of a Comparative Survey, Springer, London, UK, 2013.
UKRI: Industrial Strategy Challenge Fund: for research and innovation, available at: https://www.gov.uk/government/collections/industrial-strategy-challenge-fund-joint-research-and-innovation (last access: 28 May 2018), 2017.
UKRI: Global Challenges Research Fund, available at: https://www.ukri.org/research/global-challenges-research-fund/ (last access: 28 May 2018), 2018a.
UKRI: RCUK Policy on Open Access and Supporting Guidance, available at: https://www.ukri.org/funding/information-for-award-holders/open-access/open-access-policy/ (last access: 9 July 2018), 2018b.
Universities UK: University funding explained, Universities UK Publications, London, UK, 2016.
Vitolo, R., Stephenson, D. S., Cook, I., and Mitchell-Wallace, K.: Serial clustering of intense European storms, Meteorol. Z., 18, 411–424, https://doi.org/10.1127/0941-2948/2009/0393, 2009.
Von Peter, G., Von Dahlen, S., and Saxena, S.: Unmitigated disasters new evidence on the macroeconomic cost of natural catastrophes, BIS, Basel, Switzerland, 38 pp., 2012.
Ward, V., House, A., and Hamer, S.: Developing a framework for transferring of knowledge into action: a thematic analysis of the literature, J. Heal. Serv. Res. Policy, 14, 156–164, 2009.
- Abstract
- Introduction
- Case study: insurance sector
- Illustrative environmental scientist persona
- Methods and ethics
- Results
- Discussion
- International applicability
- A final comment: evidence of impact in practice
- Conclusions
- Data availability
- Author contributions
- Competing interests
- Acknowledgements
- References
- Supplement
user guidefor scientists and (re)insurers.
- Abstract
- Introduction
- Case study: insurance sector
- Illustrative environmental scientist persona
- Methods and ethics
- Results
- Discussion
- International applicability
- A final comment: evidence of impact in practice
- Conclusions
- Data availability
- Author contributions
- Competing interests
- Acknowledgements
- References
- Supplement